Developing a drug from idea to market is a long and expensive enterprise, taking roughly 12-15 years and costing $1 billion or more along the way. And despite the fear and skepticism surrounding artificial intelligence, new technology in the drug discovery space could speed development and prevent failures.
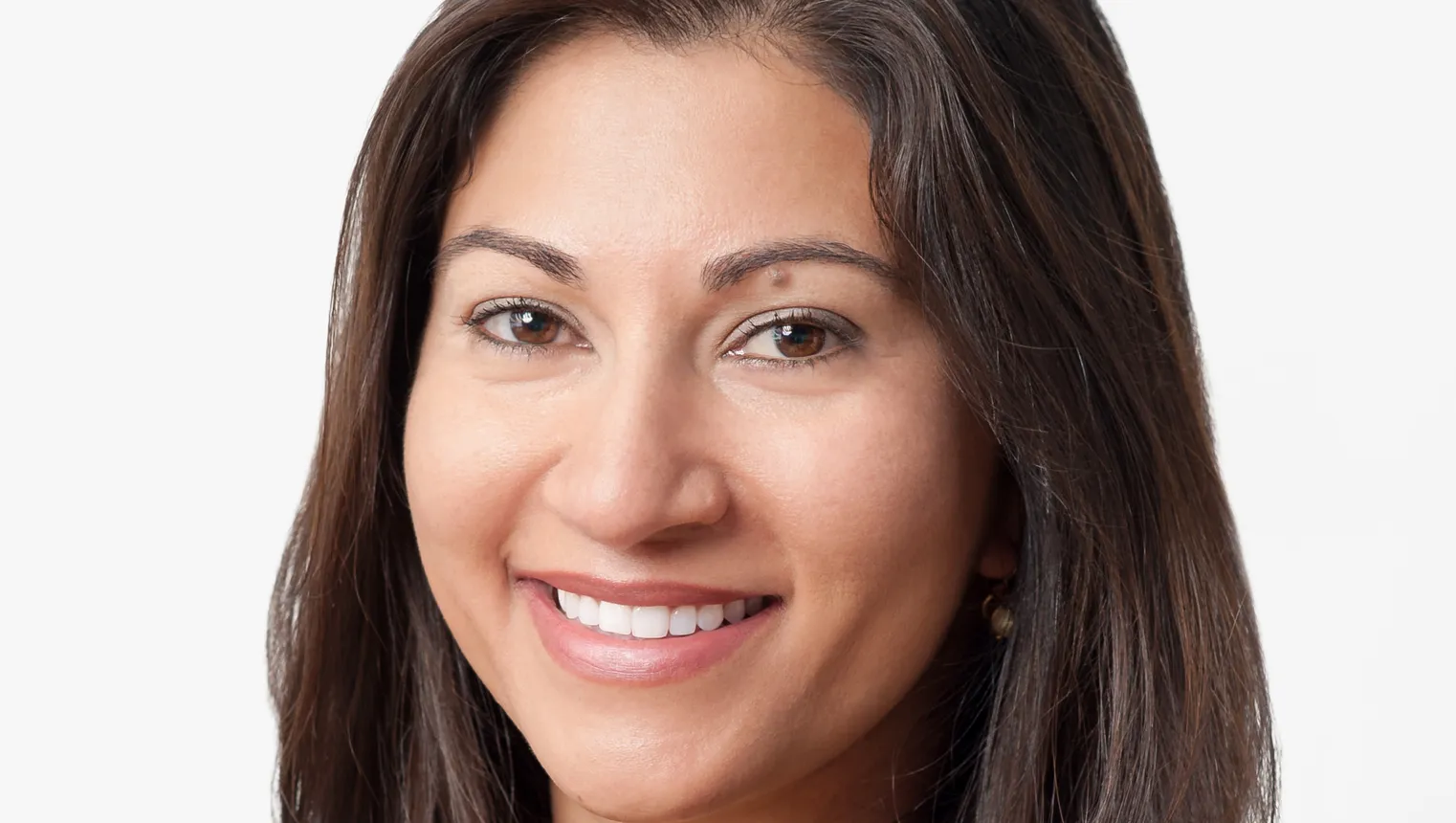
According to Shweta Maniar, global director of healthcare and life sciences at Google Cloud, AI has the potential to shave time and cost from many of those steps, such as identifying a biological target, which usually takes about a year.
“When you're talking about something that normally takes 12 months, if we can even reduce that by half or by 75%, that's pretty significant,” she said. “And multiply that by how many different targets we're looking at.”
Google Cloud is among the companies making AI products in biopharma, and it counts Pfizer, Colossal Biosciences and Cerevel Therapeutics as its early clients. Google’s tools, which it announced in May, aim to help researchers with tasks such as better identifying the function of amino acids, predicting the structure of proteins, and accelerating the discovery and interpretation of genomic data for precision treatments.
"We have to take very thoughtful steps [to ensure] it’s not just technology for the sake of technology, but it's technology for a purpose."

Shweta Maniar
Global director of healthcare and life sciences at Google Cloud
We asked Maniar, a PharmaVoice 100 honoree, to break down the use of AI in drug development — now and in the future.
The conversation has been edited for brevity and style.
PHARMAVOICE: In addition to identifying drug targets, where else in the drug development process might AI be helpful? Is there other low-hanging fruit?
SHWETA MANIAR: We see a lot of low-hanging fruit in particular areas, such as in the development of clinical trials. When you think about the application of generative AI and document generation, this is a straightforward opportunity to take this very highly manual process and help organizations increase efficiencies, and have people focus on the less-manual tasks; to be able to reallocate resources and help focus on areas where their skills can be better utilized.
Another one is around document understanding. There’s a need to understand what's happening and gather information from various structured information and unstructured information to help provide some sort of contextual understanding of [a] document and see what's in your lab notebook and in my lab notebook. We’re in the same organization — how can we compare and contrast the two?
What are some AI questions life sciences professionals ask a lot?
I think life science companies, like all verticals, are all trying to understand how you bring data into the cloud securely and safely. But there are a few differences that exist that are unique to this industry. One of the questions we always receive is, ‘What are your processes and how do you keep patient information and patient data secure?’ because that is of utmost importance. We're very proactive in sharing our approaches to build that confidence.
Another question we always receive is around trying to understand how to collaborate in this environment. They're looking for new collaboration models — how you can collaborate, what you can do with that at a massive scale. [They’re] looking for advice, input and understanding on what you can do with that information, what you can do as an organization.
Is the life sciences industry wary in any way? Why?
There are lot of questions around AI and regulation. Google believes AI is too important not to regulate. We published our own AI principles in 2018 around fairness, safety, privacy and accountability. We also shared detailed guidelines and tools to empower others on how they would use AI and other tools for social benefit.
There are a lot of concepts floating around, [but] I think we also need to ground it in the reality of not just what the technology can do, but how can the technology work within the ecosystem of the entire industry? We are seeing some very far-out, fantastic ideas of what we think generative AI could do for this industry. But we have to take very thoughtful steps [to ensure] it’s not just technology for the sake of technology, but it's technology for a purpose.