Artificial intelligence will play a role in nearly every major industry down the road, guiding logistics, decision making, creativity and more as the technology improves. In the life sciences, one of the most exciting corners where AI can drive unprecedented innovation is drug discovery; sorting through trillions of molecules and biomarkers to find the right drug for the right disease is a task these systems are profoundly suited to take on.
Beyond the public's fascination with conversational generative AI systems like ChatGPT or Google Bard, machine learning holds a great deal of potential in behind-the-scenes data processing to perform the complex tasks required to discover new compounds. The U.K.'s Exscientia and Japan's Sumitomo Dainippon Pharma were the first to bring an AI-designed drug to human trials in 2020 — showing that what normally takes about half a decade can take a computer system one year.
"In two years, it's going to be a rocket to Mars."

Kent Wakeford
Co-founder, co-CEO, Form Bio
Just over three years later, innovation in the field has led to massive leaps, like the first FDA orphan drug designation for Insilico Medicine's AI-discovered pulmonary fibrosis candidate and the first novel antibody created by Absci using generative AI. Now, more than 150 small-molecule drugs are in discovery using an AI-first approach with more than 15 in clinical trials, according to the Boston Consulting Group. The annual growth rate for that pipeline is almost 40%, and traditional pharma companies need to take the time to learn the landscape, "separating hype from actual achievement," BCG said.
"Given the transformative potential of AI, pharma companies need to plan for a future in which AI is routinely used in drug discovery," the consulting group said. "New players are scaling up fast and creating significant value, but the applications are diverse and pharma companies need to determine where and how AI can most add value for them."
The AI train has left the station, and biopharma companies need to climb aboard. But where do the tracks lead from here, and what can the industry expect along the way? One truth is certain: AI will undoubtedly play a part in the future of drug discovery and those investing early will have a leg up.
Leveraging data
Some companies in the life sciences sector are taking drug discovery down to the simplest elements using AI to mold usable compounds. For example, the New York company Ordaos specializes in creating "mini-proteins," which are biological molecules smaller than monoclonal antibodies.
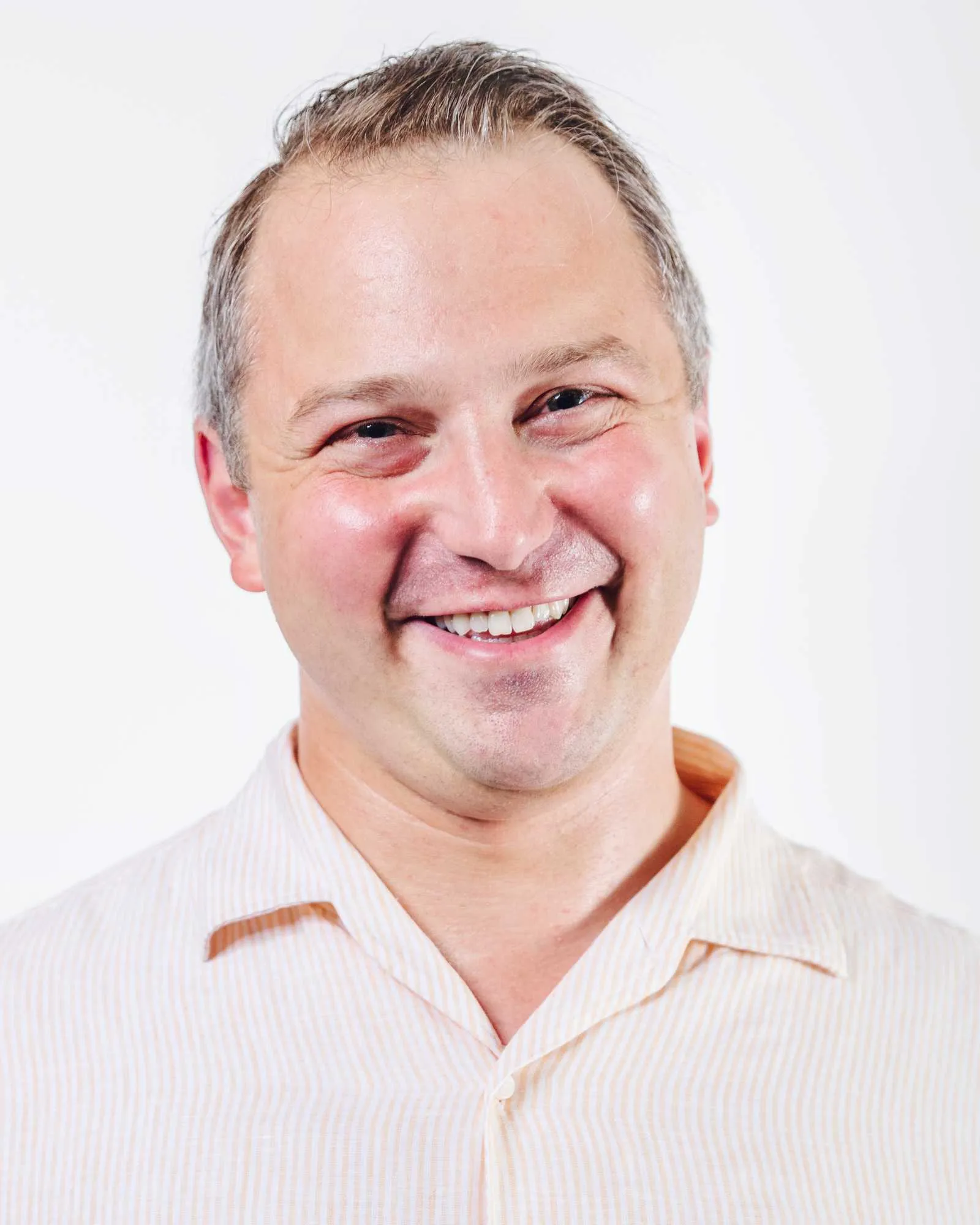
Ordaos's approach speaks to the many facets of AI in drug development and that it isn't a monolithic field with a singular approach. Whereas some AI systems are based on finding the best molecule among thousands in a more traditional discovery library, Ordaos uses a more generative angle to design a molecule rather than find it, CEO David Longo said in an interview.
"The promise of AI is we can design out whatever we want — and that's allowing us to explore more, have more diverse outputs and back train more quickly," Longo said. "The most important thing is it should allow for a reduction in the time to develop a drug from this lengthy ten-year process down to five years or less."
Ordaos, which came up in J&J's JLABS incubator and is now part of the AWS Generative AI Accelerator, is working on “mini-proteins” to design drugs for acute myeloid leukemia among other diseases.
AI is now at "an absolute inflection point," Longo said, changing the way we interface with knowledge in a fundamental way.
"There are very few times in history that we've looked at an innovation S-curve and actually watched it shift," Longo said. "From an educational standpoint, the concept of rote memorization is pretty much gone, and AI is going to provide a way of only getting to the most important pieces."
The traditional method of high-throughput screening that uses a library of thousands of molecules and tests them for stability is made almost irrelevant given AI's ability to perform the same task automatically, Longo said.
"I think in the future you're going to see far more capital-efficient discovery and design and far less medical burden as we're getting to more efficient, safer, less costly drugs with AI," Longo said.
The pharma model is still important for drug development and commercialization past the discovery stage, though, Longo said. What AI companies like Ordaos provide is leverage of data.
"AI can come in and not only offer more leads, but rather more of the right leads that are answering the right questions," Longo said. "A big topic right now is active learning, where generative AI companies can partner with pharmas to train models and provide better results more quickly."
The nature of AI is such that success only breeds more success. The models we use today will be used by tomorrow's AI to become more efficient and less dependent on human input. The risk that, for example, a toxic molecule can come about as the result of an AI discovery process is currently mitigated by control systems based on traditional knowledge, but as models evolve and learn from past iterations that risk is reduced, Longo said.
"The first model informs the second model, which informs the third, and you can have AI do anything you want," Longo said.
From discovery to drug
The discovery of a novel compound that can treat an unmet medical need is only as applicable as the ability to build and scale for wide patient use. And in an industry where manufacturing capacity has fallen behind the innovative prowess of the R&D side, AI can play a part there, as well.
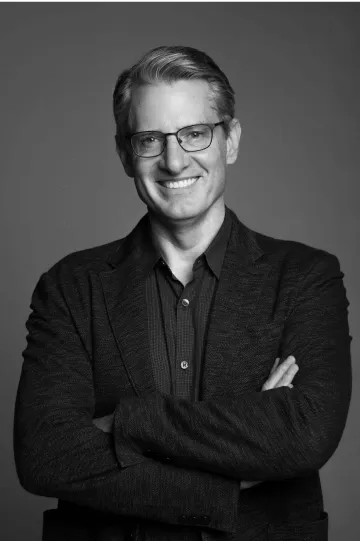
Cell and gene therapy — one of the fastest growing and tech-heavy arms of the biopharma complex — is in particular need of manufacturing solutions that start as far back as the discovery process. Kent Wakeford, co-CEO and co-founder of the AI platform company Form Bio, said that companies need the AI tools to ensure their therapies are manufacturable from the outset.
For example, a company with multiple gene therapies in their very early pipeline found that a few of the options were getting half-empty vectors, resulting in safety concerns.
"What we were able to do is understand the secondary and tertiary structures and whether the cause of truncations were chimeric or other impacts to the biomanufacturing output," Wakeford said.
And just like in pure AI drug discovery, advances in the field of supplementary platforms are self-perpetuating, Wakeford said.
"You have to train your models, and then you need to validate. We run these models in bioreactors and then take any new learnings and feed that back into the model," Wakeford said. "There are a lot of steps to building a really good AI infrastructure, but once you do it, it can grow rapidly because it's constantly learning."
The industry is coming to terms with how to approach AI, but Wakeford said that the technology is applicable in many different ways, even if risks still exist.
"There is a bit of skepticism, but also a bit of validation, which is absolutely the right thing," Wakeford said. "As these tools start to get adopted, you're going to see progress happen in different areas of a drug's lifecycle, and very quickly, from discovery to manufacturing to clinical trials to the approval process — every aspect can be impacted by AI in a different way."
No matter what the future looks like, it's coming much more quickly than it ever has, he said.
"If the industry today is like a Toyota Corolla going from point A to point B, AI is an F-1 race car, and that race car is here today," Wakeford said. "In two years, it's going to be a rocket to Mars."